Last November 4, a lecture entitled Mathematics of Deep Learning was held at the Philippe Jones Lhuillier Conference Room, 14th floor of Henry Sy Sr. Hall. The key speaker, Dr. Eduardo Mendoza—an Adjunct Professor of DLSU and the University of the Philippines Diliman (UPD), and a guest scientist in Munich, Germany—discussed the recent contributions of mathematicians to the field of deep learning (DL), an advanced independent learning done by an artificial intelligence (AI) system that provides solutions to various tasks, such as using patients’ data to recommend appropriate medicines.
DL and its historical progression
Mendoza defined data science (DS) in his lecture as the method of using statistics and machine learning (ML) to handle big data sets, remarking, “I think that is really the essence of DS, and the main component is ML.” First predicted by John W. Tukey in 1962, DS recently became in-demand for several reasons—including, for example, various companies needing information about market trends, consumers of their products, and social media engagements.
He followed with a description of ML as technology’s way of “trying to emulate the way humans learn”. “It’s a very difficult job, of course, but methods became very successful for certain tasks,” he commented, referring to the day-to-day ML applications like email filtering, voice-activated virtual personal assistants such as Siri, and search result optimization.
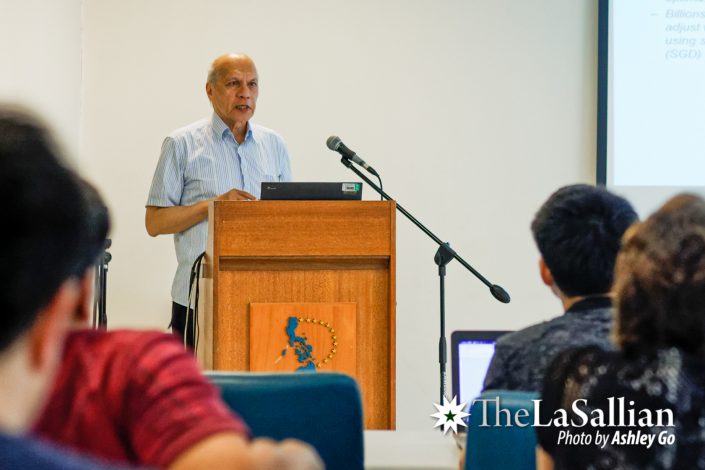
The speaker proceeded to explain DL as “ML with artificial neural networks with at least one so-called hidden layer”, pointing to its multi-layered nature that, aside from the hidden layer, consists of the input layer and the output layer. The data points first enter the input layer, then flow into the hidden layer—where necessary calculations, primarily involving weights or multipliers, are done—and eventually exit the output layer as displayed results of the performed computations.
What makes such processing powerful are the core components—deep neural networks (DNNs), which are artificial neural networks whose neurons are arranged in weight-connected layers. DNNs enable DL machines to perform a variety of tasks and have exhibited success on a variety of applications such as classifying images according to their visual content, playing various board games such as chess, and transcribing an input speech.
Filipino contributions toward DL
Mendoza recalled how his interest in DL began with a research paper about the fisherfolk communities and fish reserves in Mindoro Strait, which is a part of the Verde Island Passage and is also known as the “Center of the Center” of global marine biodiversity. “Actually, I got interested because a marine scientist at UPD, Helen Yap, sent me a preprint they had in the journal, Conservation Biology, which used DNNs based on machines,” he narrated.
In the study, DL was used to process the contents of several interviews, identifying key words concerning the economic interests of coastal communities. This enabled the researchers to better understand the delicate balance between livelihood and environment as they deliberated the possibility of temporarily changing the fishermen’s occupations in hopes of restoring Mindoro Strait’s reserves, which were being depleted by overfishing at the time.
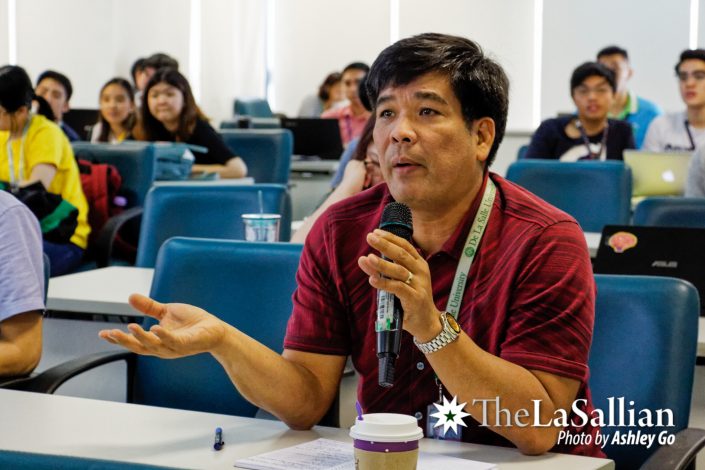
Discussing more Filipino DL applications, Mendoza cited the likes of the Fish-I System, which utilizes several cameras and a data analysis software—letting the computer perform the difficult task of sifting through what each camera captures and combining such data—in order to identify different species of fish, as well as their population count, within a given vicinity. It was developed by Dr. Laura David of the Marine Science Institute at UPD and Dr. Prospero Naval of the Computer Science Department of the same university.
Mendoza also mentioned his initiative called Machine Education Theory and Application (META), which was launched last June 12. He shared, “The idea is that you don’t [just] have ML, but you actually try to do machine teaching…You don’t just give the computer the sample data and the label, but you actually try to incorporate more knowledge from the subject matter to actually set better context for interpreting data.”
However, META’s bigger challenge is to broaden DL’s impact in the Philippines as only three major Filipino DL research groups exist so far—a group from DLSU, one from the Asian Institute of Management, and another from UPD.
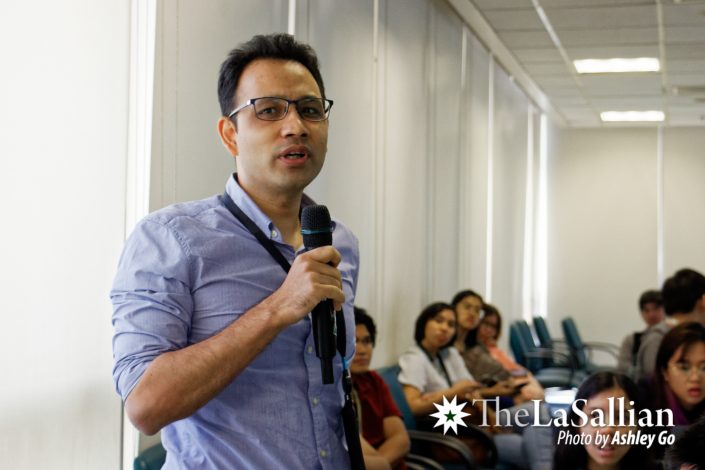
The Mathematics aspect
Mendoza tackled the emergence of Mathematics of DL (MODL) and of DS, citing some of the challenges surrounding DNNs, as these must also be built around the questions categorized under four variables: expressivity, learning, generalization, and explainability.
“Training [the machines] is more or less [a] trial-and-error [process] right now, and there is also the question [of] DNNs’ black box on the machines [as] you can’t precisely say how a prediction is achieved, and so forth,” he explained. Working with black box systems can mean getting good results, but without deeply understanding the underlying relationships and processes between the properties being modeled.
The three applications of MODL were also tackled, particularly optimal approximation, deep Taylor decomposition, and geometric deep learning.
Optimal approximation can be used to further improve the estimation of numerical or descriptive values done by DNNs through stochastic gradient descent—a method that uses one randomly selected sample to estimate the values of the larger data set.
On the other hand, Deep Taylor decomposition is a relatively new method for DNNs based on the Taylor series expansion. For example, when predicting a specific object in a digital image, the image is decomposed into input variables, forming a heatmap that specifies which pixels are necessary or most important in accurately determining the object’s identity.
Finally, geometric deep learning focuses on data analysis contained in non-Euclidean or non-flat spaces, such as graphs that have nodes acting as entities and lines serving as connections between them, and manifolds composed of points representing shapes within a space. It is used in recognizing a three-dimensional object, and in analyzing the density of social connections in networking sites like Facebook or Twitter.
Mendoza concluded the lecture by discussing the mathematics and the DL’s future altogether, “I can say that the field of deep learning has demonstrated very impressive or remarkable solutions for many tasks in science and society. The development of a robust theoretical framework to underlie this field is very important, especially [as] it becomes more and more used in critical areas of society.”