A flattened graph, whether it refers to cases, mortalities, or healthcare-related costs, means a slowdown in the spread of COVID-19. These graphs are constructed using data based on thousands of recorded cases across the country, data that is updated and published in near real-time to keep up with the spread of infection. The collection and processing of this data, and its subsequent use in models and communicative visuals such as graphs, are made possible through Statistics and Data Science.
Dr. Robert Neil Leong, an assistant professor of the Mathematics and Statistics Department and a member of the Leading Evidence-based Actions through Data Science (LEADS) for the Health Security and Resilience Consortium, describes Statistics as a field “mainly driven by the mathematics of probability or chances”. It involves proper data collection and study design, and is “closer to the scientific process in developing and interpreting models and results,” he added.
Data Science, in contrast, is “more applied”. Advancements in computational technology have made possible the processing of large volumes of “noisy data”—data sets that contain unneeded details—toward gleaning meaningful information.
Though Statistics and Data Science have different niches, they work closely together. In practice, Data Science creates close to real-time visualizations of COVID-19 data, which helps in providing situational awareness to policymakers and generating initial hypotheses. Statistics then verifies these hypotheses through the use of proper data gathering and analysis techniques as well as the development of models.
Starting point
Work begins with the collection of raw data from the Philippine healthcare system. Every day, the Department of Health (DOH) releases data drops—massive spreadsheets containing unprocessed information on tens of thousands of COVID-19 cases in the country. This information is collected from healthcare facilities, who then pass these on to higher government offices until it reaches the DOH Central Office.
Louie Dy, a member of the University of the Philippines (UP) COVID-19 Pandemic Response Team, highlights the problems inherent in this system. “The devolved nature of the Philippine health system,” he says, “creates many levels of data gathering and stewardship—hence problems in the data infrastructure.”
Each level in the process opens the data to possible modifications such that once it “reaches the DOH Epidemiology Bureau, there are definitely many errors and problems in cleaning up,” Dy adds.
Meanwhile, Leong points out underestimation issues, which he says are inherent in epidemiological data. These issues can be due to underascertainment—from some “asymptomatic or very mild” individuals who do not undergo testing—or underreporting, which can be the result of a “failure to properly report cases despite having [been] presented to a healthcare facility”.
Despite these limitations, the DOH data drop is an invaluable source of information, especially since it is free for public use. Teams of statisticians and data scientists, such as the LEADS for Health Security and Resilience Consortium and the UP COVID Response Team, have utilized the data and translated them into more digestible formats.
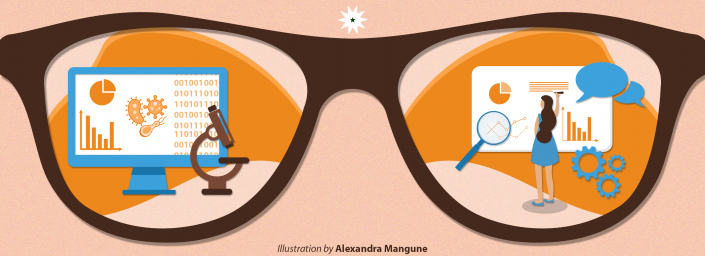
The pandemic in numbers
Aside from graphs, other statistical measures can be used to gauge the current state of the pandemic. An example of this is the effective reproductive number (Rt), which is used to describe how infectious a disease is. When Rt equals two, it means that an infected individual can pass on the disease to two more people on average. Rt dropping below one is a good indicator that there is less viral transmission.
Data can also be used to calculate estimates and predictions. In early June, the UP OCTA Research team projected a total of 40,000 cases by the end of the month and later estimated 85,000 cases by the end of July. Forecasts such as these are the result of mathematical models meant to approximate how situations may play out in reality.
Models are not expected to be perfect or exact representations of the situation, Leong says, stressing that one cannot get absolutely correct values based on models, no matter their complexity. He instead advises to “[look] after the qualitative insight of what can happen if you do this or that as produced by your models.”
“When you introduce community lockdowns in models,” he elaborates, “you do not ask how many cases there will end up [being], but rather you should be asking by how much and by when do we expect to see a decrease in growth in cases.”
For the people
“The beauty with these fields is the ability to inform,” Leong says. “As a certain saying goes, ‘All models are wrong, but [some] can still be useful’—especially if properly conceptualized. So in other words, the information by itself already makes the fields useful.” The crux, he says, lies in how to translate the information into action.
Dy sums up the goal behind the work of Statistics and Data Science in two words: risk communication. “When we present data,” he adds, “we should always make it a point that the public understands the implications and assumptions of the data, rather than making blanket statements.” He asserts that the public should see the truth and the full picture, or realize its limitations, so they can make informed decisions and navigate the risks encountered by everyone during this pandemic.
“It is important for readers and especially users of these results to be mindful about how the related data [was] were collected or generated and how the indices were measured,” Leong stresses. “One has to take the responsibility to always critically think about the results and not just take what are written [at] face value.”
The work of Dy, Leong, and others in their field is only a portion of the national COVID-19 response, a very big picture that requires interdisciplinary coordination to fully weave together their efforts. “Because it is a public crisis, all disciplines have a role. Statisticians and data scientists working with experts in other fields is always important,” Dy concludes.