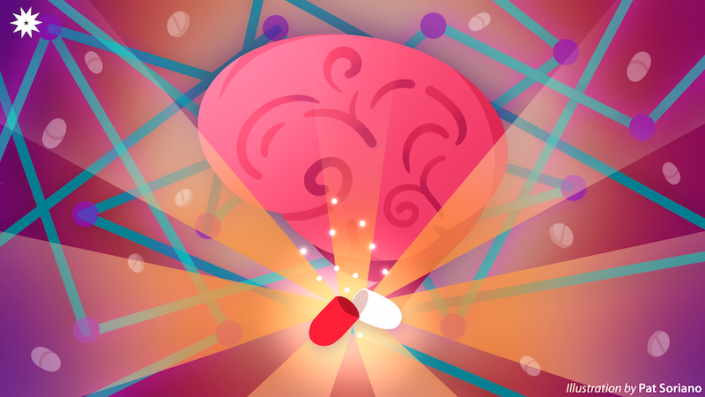
Drug design is an involved, expensive, and time-consuming process that forms the basis of modern Medicine. However, pharmaceutical scientists are now able to leverage technologies like artificial intelligence (AI) to expedite both drug design and the delivery of drugs to the market for purchase.
Capping ANN predictions
Within the broad umbrella of AI lies deep learning: a subset of machine learning that mimics the structure of the brain’s biological neural networks.
These artificial neural networks (ANNs) consist of thousands of interconnected artificial neurons called processing units, where each unit contains a weight that determines the strength of the connections between them. ANNs are composed of three layers: input, process, and output.
With the assistance of mathematical models and algorithms, each artificial neuron goes through a complicated training process to collect a series of input signals from which the collective neural network learns. In the process, these input signals are transformed into the desired output signals, which are used for the prediction stage.
“[The results] have a very high level of precision” because of the collected data, says Rennan Baldovino, an associate professor from the Manufacturing Engineering and Management Department of the University. Because of this precision, he reasons that “we can use neural networks or other prediction tools for drug discovery.” However, as precise as their predictions may be, relying on them alone can have its drawbacks.
Harnessing the brain’s analog
The growing number of COVID-19 cases has provided scientists an avalanche of information that has allowed them to leverage smart approaches to streamline the manufacturing and designing of drugs and vaccines.
“The primary strength usually in drug manufacturing is the data analysis,” Baldovino explains, adding “once you have the data, this will be used to model the performance of the creation of drugs.” This means that drug discovery and its design can now be data-driven rather than using the usual trial-and-error methods.
Nevertheless, pharmaceutical companies must conduct parallel investigations to validate data based on the prediction provided by the ANNs despite their high precision.
Using ANNs, scientists from pharmaceutical companies like Pfizer and Sanofi are boosting their efforts to develop drugs to mitigate the spread of the coronavirus.
However, entirely relying on ANNs to develop drugs and vaccines is insufficient. Since neural networks lack the general intelligence of medical practitioners, they can produce puzzling predictions that could harm patients if physicians blindly follow them. “You never know what will happen to the patient subjected to the drug,” Baldovino cautions.
He explains that although ANNs can provide 97-percent precision in their outputs, scientists should always have the final say to benefit public health.
Disclosing drug complications
While neural networks are up-and-coming, they can only predict drug discovery results. “These results aren’t going to be accepted by [the] Food and Drug Administration,” Baldovino says.
The process begins when a multitude of chemical compounds are screened for drug component candidacy. However, because there are so many compounds to screen, this is nothing short of an ongoing trial.
In their current form, ANNs are capable of accelerating the screening process. Instead of individually testing the compounds, the neural network could be fed data on both the compounds and the target disease.
In essence, ANNs could narrow down candidates for compound refinery and reduce the resources and time required for drug research. On the other hand, Baldovino discloses that one cannot expect current ANNs to replace the entirety of the drug design process—to do so is a fault.
Baldovino reminds that age, gender, and blood type are some of the uncontrolled factors which ANNs are currently incapable of considering. These are factors that carry massive weight in drug delivery.
“During the clinical testing, you’re not allowed to use predictions,” the professor declares. Therefore, instead of predicting test results, ANNs analyze the symptoms a patient experiences after the drug administration.
Recurrent applications
Aside from their drug design applications, Baldovino mentions that ANNs have also been used to detect the probability of having specific side effects and symptoms of those inoculated with the COVID-19 vaccine months prior.
A similar approach was adopted in the United States, where ANNs were applied to optimize vaccine distribution by developing a sequence-learning model to track and predict the behavior of the pandemic across their country. ANNs have also been crucial for the development of medical support devices and prostheses for amputees.
“It’s difficult for [amputees] to control and maneuver the wheelchair,” Baldovino comments, touting that the incorporation of ANNs in wheelchairs will make for a more streamlined experience. “There’s no need to use the remote control…You just have to think that you want to move forward, left or right or that you want to stop,” he explains.
Leading aspirations
Like all many new technologies, ANNs face difficulty in breaking through different industries—particularly in healthcare. But the professor cites Robotics, Biomedical Engineering, and earthquake predictions as areas where ANNs have already been applied.
Concerning more complex and abstract issues such as drug design, where data is not available for accessible collection, the professor furthers, “I believe the use of ANN could solve some of these [nonlinear] problems.”
With illnesses, such as COVID-19, constantly evolving faster than the human brain can process, ANNs are believed to be the hope of patients in need of immediate and currently unavailable medicinal care. And as researchers aim to realize the full potential of ANNs, reaching the future in the present may not be so impossible.